Ciwan Ceylan
Grettings, and a warm welcome to my research profile!
I am an industrial PhD student at KTH Royal Institute of Technology and a member of the Wallenberg Artificial Intelligence, Autonomous Systems and Software Program (WASP), supported by SEB. My thesis, co-supervised by Professor Danica Kragic at KTH and Dr. Kambiz Ghoorchian at SEB, focuses on unsupervised representation learning for large financial transaction graphs.
My research centers on scalable methods for learning node embeddings that capture critical features like edge directionality and edge flow, both essential for modeling financial transaction networks. By developing techniques that incorporate these characteristics, I aim to enhance anomaly detection systems used in the fight against money laundering and terrorist financing, providing graph-informed insights to identify suspicious activities.
Beyond financial transaction graphs, the unsupervised learning techniques I develop have broad applicability. I explore their potential in various graph-related tasks, including node classification, graph alignment, link prediction, node clustering, and network flow prediction. I am also passionate about unsupervised learning methods beyond graph data, and I have previously published a paper on contrastive learning for image data as part of my master’s thesis.
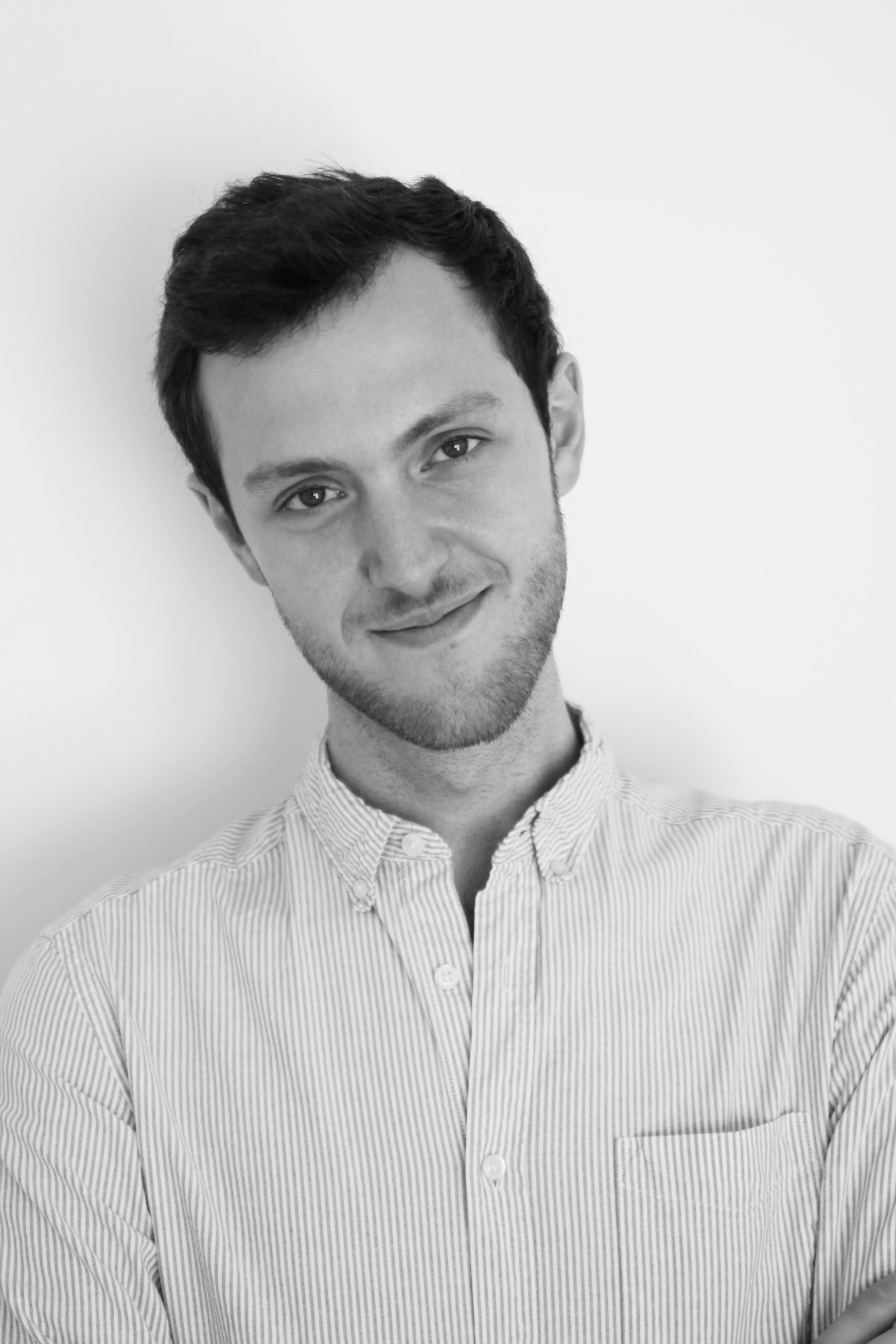
ciwan [at] kth.se
Stockholm, Sweden